AI predicts extensive material properties to break down a previously insurmountable wall
If the properties of materials can be reliably predicted, then the process of developing new products for a huge range of industries can be streamlined and accelerated. In a study published in Advanced Intelligent Systems, researchers from The University of Tokyo Institute of Industrial Science used core-loss spectroscopy to determine the properties of organic molecules using machine learning.
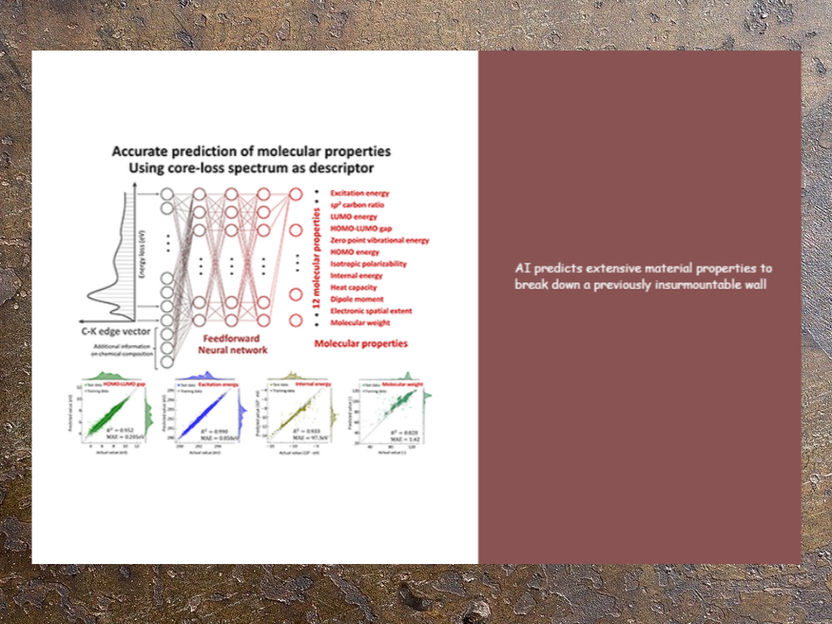
Researchers from The University of Tokyo Institute of Industrial Science use a machine learning approach to successfully predict material properties that have never before been determined
Institute of Industrial Science, the University of Tokyo
The spectroscopy techniques energy loss near-edge structure (ELNES) and X-ray near-edge structure (XANES) are used to determine information about the electrons, and through that the atoms, in materials. They have high sensitivity and high resolution and have been used to investigate a range of materials from electronic devices to drug delivery systems.
However, connecting spectral data to the properties of a material—things like optical properties, electron conductivity, density, and stability—remains ambiguous. Machine learning (ML) approaches have been used to extract information for large complex sets of data. Such approaches use artificial neural networks, which are based on how our brains work, to constantly learn to solve problems. Although the group previously used ELNES/XANES spectra and ML to find out information about materials, what they found did not relate to the properties of the material itself. Therefore, the information could not be easily translated into developments.
Now the team has used ML to reveal information hidden in the simulated ELNES/XANES spectra of 22,155 organic molecules. “The ELNES/XANES spectra of the molecules, or their “descriptors” in this scenario, were then input into the system,” explains lead author Kakeru Kikumasa. “This descriptor is something that can be directly measured in experiments and can therefore be determined with high sensitivity and resolution. This method is highly beneficial for materials development because it has the potential to reveal where, when, and how certain material properties arise.”
A model created from the spectra alone was able to successfully predict what are known as intensive properties. However, it was unable to predict extensive properties, which are dependent on the molecular size. Therefore, to improve the prediction, the new model was constructed by including the ratios of three elements in relation to carbon (which is present in all organic molecules) as extra parameters to allow extensive properties such as the molecular weight to be correctly predicted.
“Our ML learning treatment of core-loss spectra provides accurate prediction of extensive material properties, such as internal energy and molecular weight. The link between core-loss spectra and extensive properties has previously never been made; however, artificial intelligence was able to unveil the hidden connections. Our approach might also be applied to predict the properties of new materials and functions” says senior author Teruyasu Mizoguchi. “We believe that our model will be a very useful tool for the high-throughput development of materials in a wide range of industries.”
Original publication
Other news from the department science
Most read news
More news from our other portals
See the theme worlds for related content
Topic World Spectroscopy
Investigation with spectroscopy gives us unique insights into the composition and structure of materials. From UV-Vis spectroscopy to infrared and Raman spectroscopy to fluorescence and atomic absorption spectroscopy, spectroscopy offers us a wide range of analytical techniques to precisely characterize substances. Immerse yourself in the fascinating world of spectroscopy!
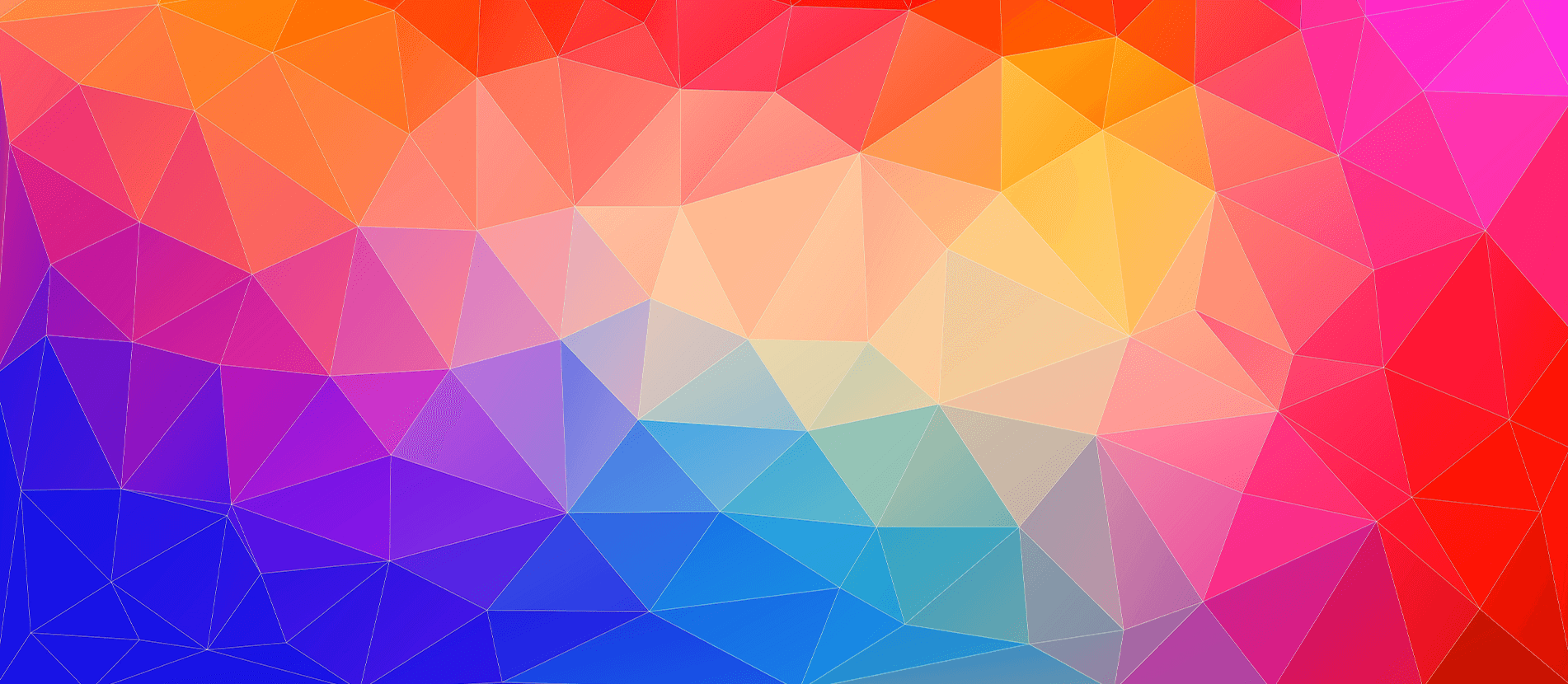
Topic World Spectroscopy
Investigation with spectroscopy gives us unique insights into the composition and structure of materials. From UV-Vis spectroscopy to infrared and Raman spectroscopy to fluorescence and atomic absorption spectroscopy, spectroscopy offers us a wide range of analytical techniques to precisely characterize substances. Immerse yourself in the fascinating world of spectroscopy!