Innovative solutions for chemical challenges: Harnessing the potential of machine learning
Revolutionising chemical research with AI?
In a review published in Engineering, scientists explore the burgeoning field of machine learning (ML) and its applications in chemistry. Titled “Machine Learning for Chemistry: Basics and Applications,” this comprehensive review aims to bridge the gap between chemists and modern ML algorithms, providing insights into the potential of ML in revolutionizing chemical research.
Over the past decade, ML and artificial intelligence (AI) have made remarkable strides, bringing us closer to the realization of intelligent machines. The advent of deep learning methods and enhanced data storage capabilities has played a pivotal role in this progress. ML has already demonstrated success in domains such as image and speech recognition, and now it is gaining significant attention in the field of chemistry, which is characterized by complex data and diverse organic molecules.
However, chemists often face challenges in adopting ML applications due to a lack of familiarity with modern ML algorithms. Chemistry datasets typically exhibit a bias towards successful experiments, while a balanced perspective necessitates the inclusion of both successful and failed experiments. Furthermore, incomplete documentation of synthetic conditions in literature poses additional challenges. Computational chemistry, where datasets can be reliably constructed from quantum mechanics calculations, has embraced ML applications more readily. Nonetheless, chemists need a basic understanding of ML to harness the potential of data recording and ML-guided experiments.
This review serves as an introductory guide to popular chemistry databases, two-dimensional (2D) and three-dimensional (3D) features used in ML models, and popular ML algorithms. It delves into three specific chemistry fields where ML has made significant progress: retrosynthesis in organic chemistry, ML-potential-based atomic simulation, and ML for heterogeneous catalysis. These applications have either accelerated research or provided innovative solutions to complex problems. The review concludes with a discussion of future challenges in the field.
The rapid advancement of computing facilities and the development of new ML algorithms indicate that even more exciting ML applications are on the horizon, promising to reshape the landscape of chemical research in the ML era. While the future is difficult to predict in such a fast-evolving field, it is undeniable that the development of ML models will lead to enhanced accessibility, generality, accuracy, intelligence, and ultimately, higher productivity. The integration of ML models with the Internet offers a promising avenue for sharing ML predictions worldwide.
However, the transferability of ML models in chemistry poses a common challenge due to the diverse element types and complex materials involved. Predictions often remain limited to local datasets, resulting in decreased accuracy beyond the dataset. To address this issue, new techniques such as the global neural network (G-NN) potential and improved ML models with more fitting parameters are being explored. While ML competitions in data science have produced exceptional algorithms, there is a need for more open ML contests in chemistry to nurture young talent.
Excitingly, end-to-end learning, which generates final output from raw input rather than designed descriptors, holds promise for more intelligent ML applications. AlphaFold2, for example, utilizes the one-dimensional (1D) structure of a protein to predict its 3D structure. Similarly, in the field of heterogeneous catalysis, an end-to-end AI model has successfully resolved reaction pathways. These advanced ML models can also contribute to the development of intelligent experimental robots for high-throughput experiments.
As the field of ML continues to evolve rapidly, it is crucial for chemists and researchers to stay informed about its applications in chemistry. This review serves as a valuable resource, providing a comprehensive overview of the basics of ML and its potential in various chemistry domains. With the integration of ML models and the collective efforts of the scientific community, the future of chemical research holds immense promise.
Original publication
Other news from the department science
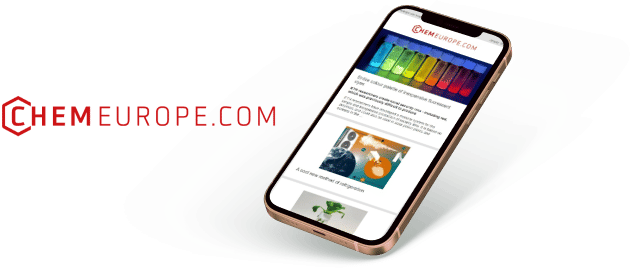
Get the chemical industry in your inbox
By submitting this form you agree that LUMITOS AG will send you the newsletter(s) selected above by email. Your data will not be passed on to third parties. Your data will be stored and processed in accordance with our data protection regulations. LUMITOS may contact you by email for the purpose of advertising or market and opinion surveys. You can revoke your consent at any time without giving reasons to LUMITOS AG, Ernst-Augustin-Str. 2, 12489 Berlin, Germany or by e-mail at revoke@lumitos.com with effect for the future. In addition, each email contains a link to unsubscribe from the corresponding newsletter.
Most read news
More news from our other portals
Last viewed contents
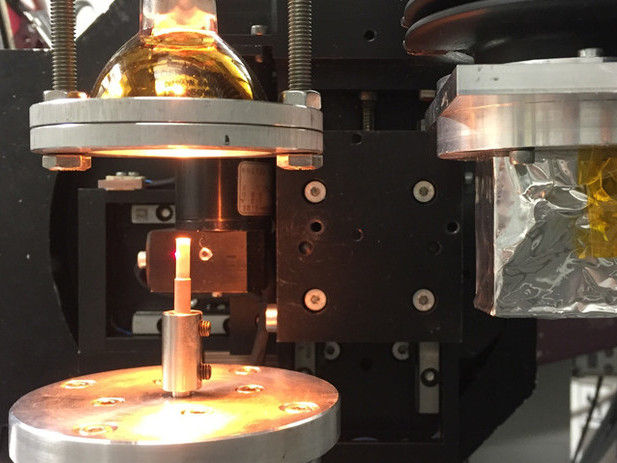
Tomoscopy: new imaging method - Thanks to a world record in tomography, synchrotron radiation can be used to watch how metal foam forms
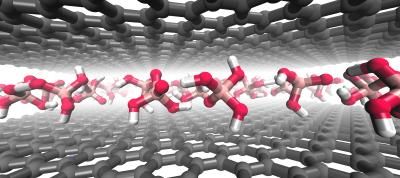
Rethinking the basic science of graphene synthesis - Discovery shows route to super-strong material's industrial-scale production
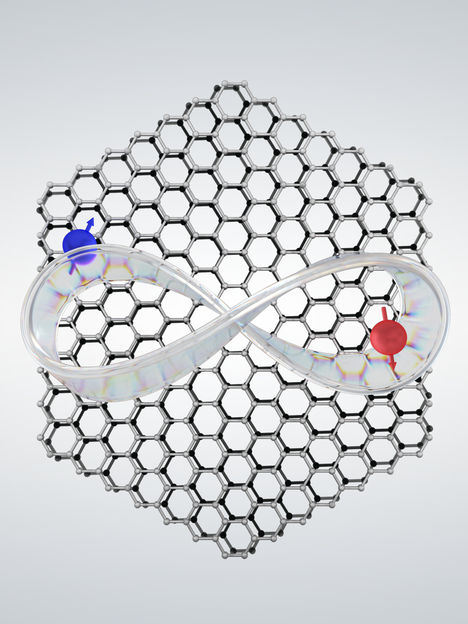
The interplay between Topology and Magnetism has a bright future
Haplogroup_J_(Y-DNA)
Howard_Malmstadt
Americaine
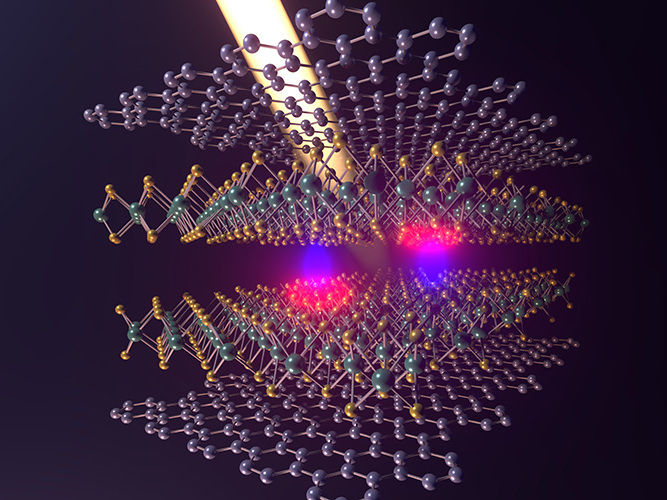
A highly light-absorbent and tunable material - New approach to developing optoelectronic devices
Arkema: Price increase of Durastrength, Plastistrength and Clearstrength
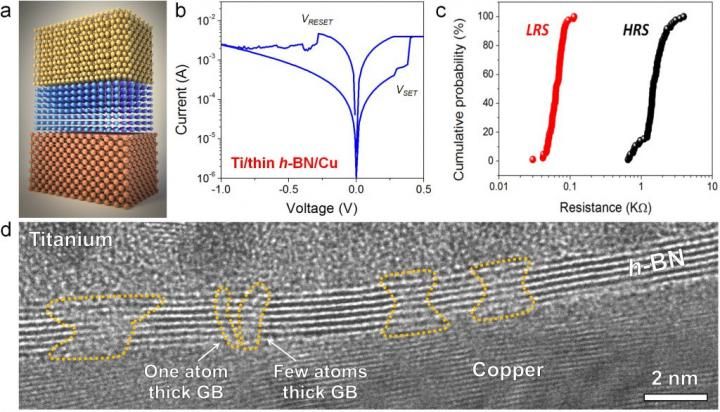