Thanks to machine learning, the future of catalyst research is now!
New study defies conventions and proposes a technology-driven method to identify useful catalyst combinations
To date, research in the field of combinatorial catalysts has relied on serendipitous discoveries of catalyst combinations. Now, scientists from Japan have streamlined a protocol that combines random sampling, high-throughput experimentation, and data science to identify synergistic combinations of catalysts. With this breakthrough, the researchers hope to remove the limits placed on research by relying on chance discoveries and have their new protocol used more often in catalyst informatics.
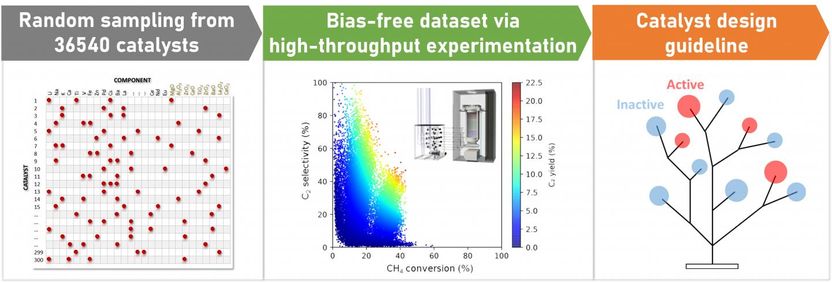
300 quaternary catalysts are randomly sampled from a large material space, where their performance in relation to OCM is systematically evaluated by high-throughput experimentation, followed by machine learning, to identify a bias-free dataset in order to learn the underlying patterns in catalyst performance which are eventually used for further catalyst discoveries.
JAIST
Catalysts, or their combinations, are compounds that significantly lower the energy required to drive chemical reactions to completion. In the field of "combinatorial catalyst design," the requirement of synergy--where one component of a catalyst complements another--and the elimination of ineffective or detrimental combinations are key considerations. However, so far, combinatorial catalysts have been designed using biased data or trial-and-error, or serendipitous discoveries of combinations that worked. A group of researchers from Japan has now sought to change this trend by trying to devise a repeatable protocol that relied on a screening instrument and software-based analysis.
Their new study, published in ACS Catalysis, details the identification of effective catalyst combinations, using the proposed protocol, for the oxidative coupling of methane (OCM). OCM is a widely used chemical reaction used to convert methane into useful gases in the presence of oxygen and the catalyst. Elaborating on the motivations behind the study, Dr. Toshiaki Taniike, Professor at the School of Materials Science, Japan Advanced Institute of Science and Technology and corresponding author of the study, says, "Combinatorial catalyst design is hardly generalizable, and the empirical aspect of the research has biased the literature data toward accidentally found combinations".
To derive a bias-free dataset from OCM for devising the protocol, the researchers sampled randomly 300 solid catalysts from a vast materials space containing upwards of 36,000 catalysts! Screening such a large number of catalysts is near impossible by human standards. Hence, the team used a high-throughput screening instrument to evaluate their performance at facilitating OCM. The obtained dataset was used to outline the novel protocol, aimed at providing a guideline for catalyst design. This was implemented in the form of a "decision tree classification," which is a form of machine learning that helped in understanding the efficiency of the selected catalyst combinations, in giving better OCM yield. This, in turn, helped in drawing up the required catalyst design guidelines.
Interestingly, the results showed that, even with random sampling, 51 out of the 300 catalysts gave a better OCM yield when compared to the alternative non-catalytic process. Explaining the potential implications of their discovery, Dr. Keisuke Takahashi, Associate Professor at Hokkaido University and co-author of this study, says, "The combination of high throughput experimentation and data science has already demonstrated the power of bias-free catalyst big data in finding novel catalysts as well as a catalyst design guideline. It is also important to state the essentiality of these approaches for implementing such a demanding study in a realistic time frame. By equipping all the essential techniques of the study, truly nonempirical catalyst developments could be realized".
Indeed, we can hope, along with the scientists, that this strategy will "catalyze" several future material science discoveries!
Original publication
Other news from the department science
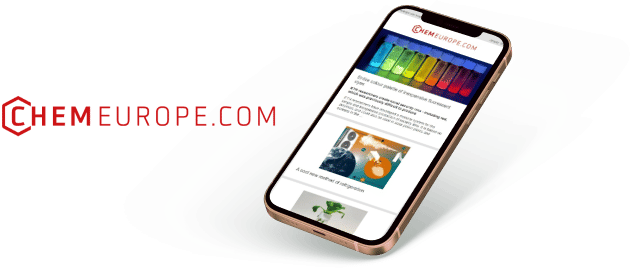
Get the chemical industry in your inbox
By submitting this form you agree that LUMITOS AG will send you the newsletter(s) selected above by email. Your data will not be passed on to third parties. Your data will be stored and processed in accordance with our data protection regulations. LUMITOS may contact you by email for the purpose of advertising or market and opinion surveys. You can revoke your consent at any time without giving reasons to LUMITOS AG, Ernst-Augustin-Str. 2, 12489 Berlin, Germany or by e-mail at revoke@lumitos.com with effect for the future. In addition, each email contains a link to unsubscribe from the corresponding newsletter.
Most read news
More news from our other portals
Last viewed contents
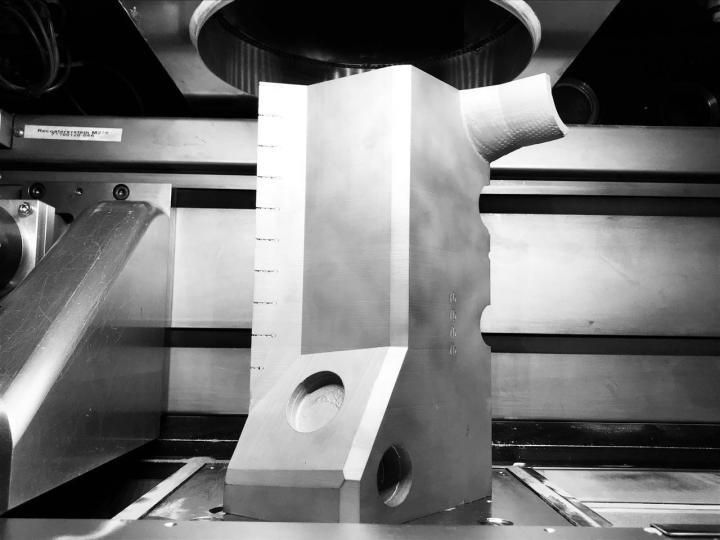
3-D printed metals can be both - strong and ductile
Puddling_(metallurgy)
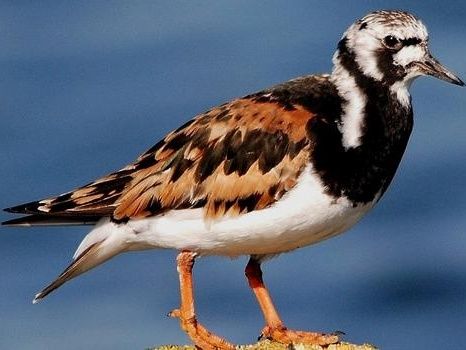
PFAS: Found 180 times more ‘forever chemicals’ in birds - New technology allows for much more precise measurements of these chemicals in organisms
Analytik Jena Closed Third Quarter Below Expectations
Cinoxacin
Yuzpe_regimen
Hollow_moon
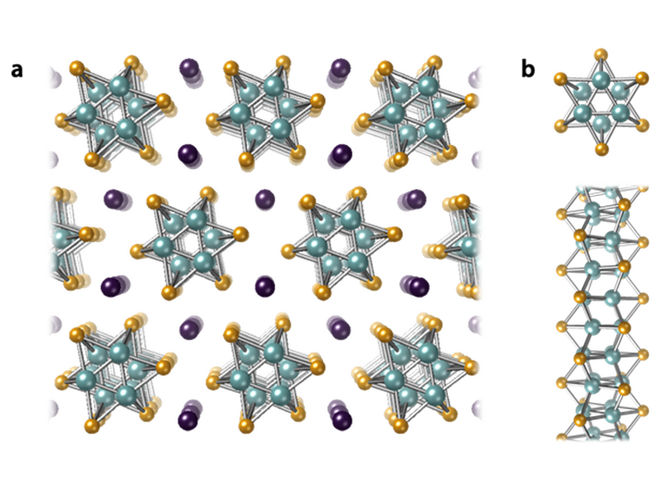
Scientists thread rows of metal atoms into nanofiber bundles - Intercalation of indium into nanostructures promises applications to nanocircuitry
Category:Intracellular_receptors
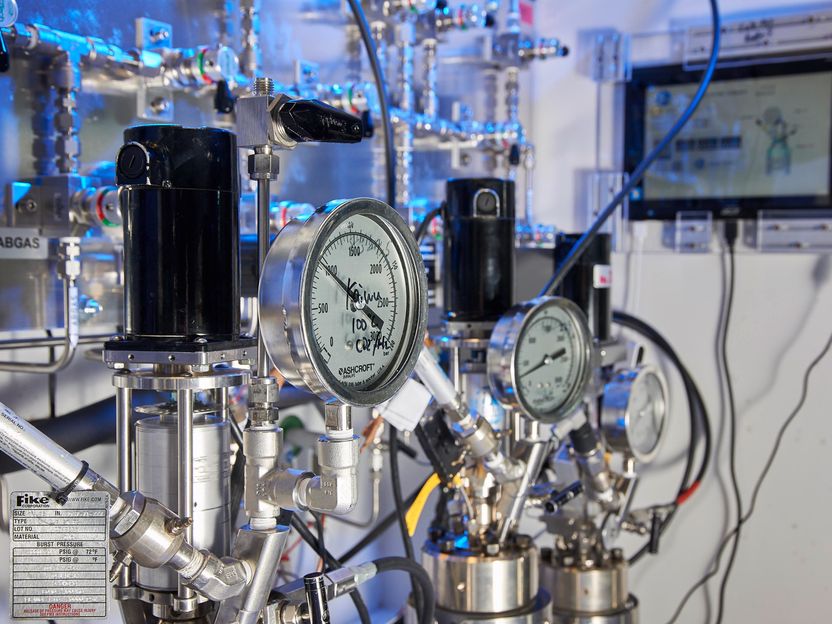